Ideal Tips About What Are Smoothing Techniques R Ggplot Dashed Line
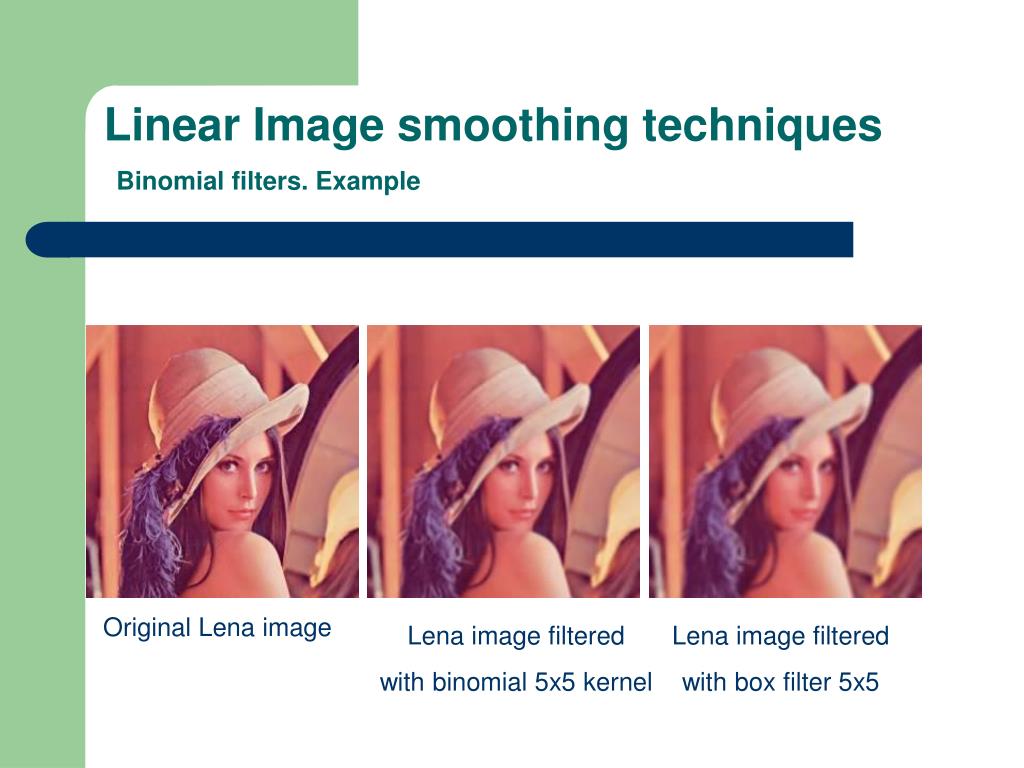
Smoothing techniques are kinds of data preprocessing techniques to remove noise from a data set.
What are smoothing techniques. Smoothing is usually done to help us better see patterns, trends for example, in time series. The histogram is a familiar type of plot that uses smoothing. In this paper, we review the statistical.
Data smoothing can be defined as a statistical approach of eliminating outliers from datasets to make the patterns more noticeable. Tararua district council could opt for a “smoothing” of rates to defer a portion of a pending 16.29% hike. Smoothing methods often have an associated tuning parameter which is used to control the extent of smoothing.
The random method, simple moving average, random walk, simple exponential, and exponential moving average are some of the methods used for data smoothing. It is accomplished by using algorithms that remove. Smoothing here means that we cannot differentiate the location of individual points in a bin:
This allows important patterns to more clearly stand out. Introducing the whittaker smoother, otherwise known as the perfect smoother. Smoothing refers to looking at the underlying pattern of a set of data to establish an estimate of future values.
In this post, you will go through a quick introduction to various different smoothing techniques used in nlp in addition to related formulas and examples. This results in an output that reveals underlying trends, patterns, or cyclical components more clearly, thereby improving the quality of the data for subsequent analysis. Smoothing can be achieved through a range of different techniques, including the use of the average function and the exponential smoothing formula.
Other names given to this technique are curve fitting and low pass filtering. There are 3 different smoothing methods to use in different 3 cases: This often involves broadening the distribution by redistributing weight from high probability regions to zero probability regions.
The aim of smoothing is to give a general idea of relatively slow changes of value with little attention paid to the close matching of data values, while curve fitting concentrates on achieving as close a match as possible. Exponential and moving average are relatively simple smoothing techniques and should not be performed on data sets involving seasonality. Data smoothing techniques are used to remove noise and variability from data sets to uncover underlying patterns and trends.
A histogram aggregates data values by putting points into bins and plotting one bar for each bin. Data smoothing can be used to help. Exponential smoothing is a method of forecasting that uses a weighted average of past observations to predict future values.
When the sharpness is turned up. One common smoothing technique used in economic research is seasonal adjustment. Data smoothing in data science is a statistical technique for removing outliers from datasets so that patterns can be seen more clearly.
It is designed to detect trends in the presence of noisy data in cases in which the shape of the trend is unknown. Insanely fast smoothing and interpolation in just a few lines of python or rust code Data smoothing is a statistical technique used to remove noise or irregularities from a dataset, resulting in a smoother representation of the underlying trend or pattern.